In the rapidly evolving business landscape of 2024, innovation is not just a competitive advantage but a necessity. However, with the high rewards of innovation come inherent risks—misjudging market trends, overlooking consumer demands, or falling behind in technology can lead to significant setbacks. As companies strive to navigate these uncertainties, the role of data analytics becomes crucial in managing innovation risk. By transforming vast amounts of data into actionable insights, businesses can not only anticipate changes but also make informed decisions that align with both current market dynamics and future projections.
One of the core strategies involves employing predictive analytics to decode market trends and consumer behavior, allowing companies to stay ahead of the curve. Real-time data monitoring and response systems further empower businesses to act swiftly and effectively, adjusting their strategies in response to live information. The integration of AI and machine learning into decision-making processes offers another layer of sophistication, enabling more accurate predictions and smarter risk management. Moreover, developing robust data-driven risk assessment models helps in quantifying the risks associated with potential innovations and determining the best courses of action. Lastly, as data becomes a cornerstone of strategic decision-making, maintaining ethical and secure data management practices is paramount to preserve trust and comply with increasing regulatory requirements. This article delves into each of these subtopics, exploring how businesses can leverage data analytics to not only mitigate innovation risks but also drive success in the unpredictable year of 2024.
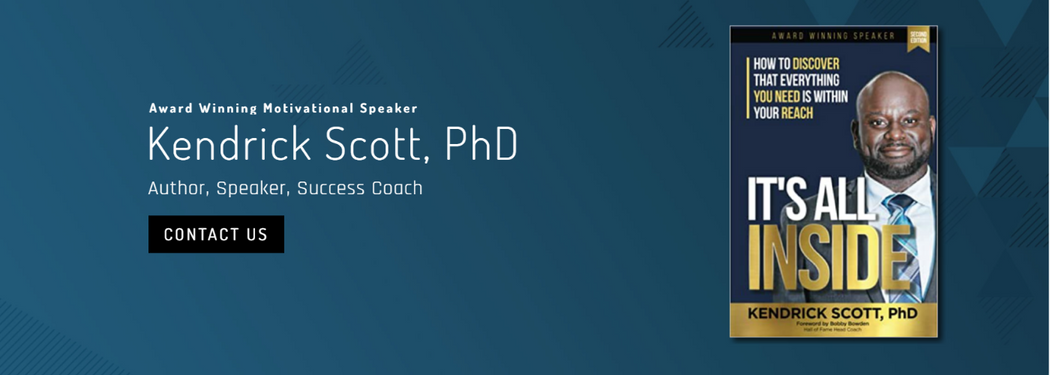
Predictive Analytics for Market Trends and Consumer Behavior
Predictive analytics stands as a pivotal tool for businesses aiming to manage innovation risk effectively, especially in the evolving landscape of 2024. By harnessing the power of predictive analytics, companies can gain foresight into market trends and shifts in consumer behavior, thereby allowing them to make informed decisions that align with future market demands.
The essence of predictive analytics lies in its ability to process and analyze vast amounts of data to forecast future events based on historical and current data trends. For instance, by examining data from social media, customer reviews, and purchase histories, businesses can predict which products will see increased demand or what new services consumers might expect. Such insights enable businesses to innovate proactively rather than reactively, reducing the risks associated with launching new products or entering new markets.
Moreover, predictive analytics helps companies in identifying potential market disruptions before they fully manifest. This early-warning system enables businesses to adjust their strategies, mitigate risks, and capitalize on emerging opportunities. In a fast-paced and competitive environment like that of 2024, being able to predict and adapt to market dynamics is crucial for sustaining innovation and maintaining a competitive edge.
Furthermore, understanding consumer behavior through predictive analytics can lead to more personalized customer experiences, which are critical for customer retention and satisfaction. As businesses look to innovate, maintaining a strong connection with the consumer base through tailored experiences and products can significantly reduce the uncertainty and risk associated with innovation.
In summary, predictive analytics serves as a cornerstone for businesses aiming to manage innovation risk in 2024 by offering valuable insights into market trends and consumer behavior, thus enabling companies to stay ahead of the curve and make data-driven decisions that bolster their innovation strategies.
Real-time Data Monitoring and Response Systems
Real-time data monitoring and response systems are crucial for businesses aiming to leverage data analytics to manage innovation risk in 2024. These systems enable organizations to track and analyze data as it is generated, providing immediate insights that can be vital for making informed decisions quickly. This capability is particularly important in a rapidly changing market environment where delays in responding to new information can result in missed opportunities or heightened risks.
Implementing real-time data systems allows companies to monitor various aspects of their operations and market conditions continuously. For example, they can track consumer reactions to a new product launch instantly or monitor supply chain disruptions as they happen. This immediate feedback loop helps businesses to respond proactively to potential issues before they escalate, thus minimizing risk and enhancing operational efficiency.
In addition, real-time data systems facilitate a more dynamic and agile approach to innovation. Companies can test new ideas and innovations in real-time, adjusting their strategies based on immediate data-driven feedback. This approach not only reduces the risks associated with new initiatives but also increases the speed of innovation, allowing companies to stay ahead of the competition.
Furthermore, as we move into 2024, the integration of advanced technologies such as IoT (Internet of Things) and edge computing with real-time data systems will likely enhance the ability of businesses to gather and analyze data from a broader range of sources. This integration will provide deeper insights and a more comprehensive understanding of the operational and market landscape, further aiding in effective risk management and decision-making processes.
Integration of AI and Machine Learning in Decision-Making Processes
In the context of managing innovation risk, the integration of artificial intelligence (AI) and machine learning (ML) into decision-making processes stands as a crucial strategy for businesses in 2024. As companies navigate through an increasingly data-driven era, the ability to analyze vast amounts of data and derive actionable insights is essential. AI and ML technologies are pivotal in parsing through complex datasets, identifying patterns, and predicting outcomes, thus enabling more informed and strategic decision-making.
AI and ML can dramatically enhance the accuracy of forecasts and models used in innovation. By leveraging these technologies, businesses can better anticipate market demands, optimize product development, and avoid potential pitfalls. For instance, AI algorithms can simulate various market scenarios and innovation outcomes to provide decision-makers with a risk assessment that is much more dynamic and nuanced than traditional methods.
Moreover, the use of AI and ML can streamline the decision-making process by automating routine tasks, thereby freeing up human resources to focus on more strategic aspects of innovation. This not only increases efficiency but also reduces the likelihood of human error. Furthermore, AI and ML can facilitate real-time decision-making. By continuously learning from new data, these systems can quickly adapt to changes, allowing businesses to remain agile and competitive.
In summary, the integration of AI and ML into decision-making processes is not just about enhancing the efficiency and effectiveness of these processes. It also plays a critical role in reducing innovation risk by providing businesses with the tools needed to make more informed, accurate, and timely decisions. As we move into 2024, this integration will be pivotal for businesses aiming to maintain a competitive edge in a rapidly evolving market landscape.
Data-Driven Risk Assessment Models
Data-driven risk assessment models are essential tools for businesses looking to leverage data analytics to manage innovation risk in 2024. These models use large sets of historical and real-time data to identify, evaluate, and prioritize risks associated with new business initiatives and technological innovations. By integrating diverse data sources, including market trends, consumer behavior, operational data, and external environmental factors, companies can develop sophisticated models that provide deep insights into potential risks.
The effectiveness of data-driven risk assessment models lies in their ability to quantify risks and predict their impacts on specific projects or across the business as a whole. This enables decision-makers to make more informed choices about where to allocate resources and how to mitigate potential negative outcomes. Furthermore, these models can be continuously updated with new data, allowing businesses to adapt their strategies in response to emerging risks or unexpected market changes.
Another advantage of using data-driven approaches in risk management is the potential for automation. Automated risk assessment tools can process vast amounts of data at speeds far beyond human capabilities, providing real-time risk assessments that are crucial for managing fast-paced innovation cycles. This real-time capability not only helps in identifying and responding to immediate risks but also in maintaining a proactive approach to risk management.
As we move into 2024, businesses that effectively implement and utilize data-driven risk assessment models will likely find themselves better equipped to handle the uncertainties inherent in innovation. This will not only protect them against potential failures but also position them to capitalize on opportunities more swiftly and confidently. Hence, mastering these models should be a priority for any organization aiming to thrive in the dynamically changing business environments of the future.
Ethical and Secure Data Management Practices
In the context of leveraging data analytics to manage innovation risk in 2024, ethical and secure data management practices play a crucial role. As businesses increasingly rely on data to make informed decisions, the importance of handling this data responsibly cannot be overstated. Ethical data management ensures that the company respects the privacy and rights of individuals, which in turn helps in maintaining public trust and compliance with legal standards.
Secure data management is equally important as it protects the data from unauthorized access, breaches, and losses. This is particularly vital when dealing with sensitive information that could potentially be exploited for malicious purposes. Implementing robust security measures such as encryption, access controls, and regular audits will be essential to safeguard the integrity and confidentiality of data.
Furthermore, as innovation often involves exploring new territories and technologies, companies must be vigilant about the emerging risks associated with these ventures. Ethical and secure data management practices not only help in mitigating these risks but also assist in establishing a strong foundation for sustainable innovation. By prioritizing these practices, businesses can ensure that they not only stay ahead in the competitive market but also maintain their reputation and consumer trust in the long run.
Leave a Reply