In today’s fast-paced and data-driven world, businesses across various sectors are increasingly turning to data analytics to enhance their operational efficiency. Data analytics, the science of analyzing raw data to make conclusions about that information, plays a pivotal role in optimizing operations, reducing costs, and improving overall business performance. By leveraging vast amounts of data, companies can gain insightful, actionable information that drives smarter decision-making and strategic operational improvements. This article delves into the critical role of data analytics in boosting operational efficiency across five key areas: predictive maintenance, real-time data monitoring, process optimization, supply chain management, and customer experience analysis.
Firstly, predictive maintenance utilizes data analytics to anticipate equipment failures before they occur, thus reducing downtime and maintenance costs. This proactive approach not only extends the lifespan of machinery but also enhances productivity by minimizing unplanned disruptions. Secondly, real-time data monitoring allows businesses to track operations as they happen, enabling immediate responses to any deviations from the norm and facilitating a more dynamic decision-making process. Thirdly, process optimization uses data to streamline operations, improve quality, and increase output, ensuring that businesses can achieve more with less.
Moving forward, effective supply chain management, powered by data analytics, ensures that enterprises can predict demand more accurately, manage inventory levels efficiently, and mitigate risks associated with supply chain volatility. Lastly, customer experience analysis helps companies understand customer behaviors and preferences, leading to tailored services and products, thereby boosting customer satisfaction and loyalty. Throughout this article, we will explore how these facets of data analytics contribute to enhanced operational efficiency, providing a comprehensive understanding of its transformative impact on modern business practices.
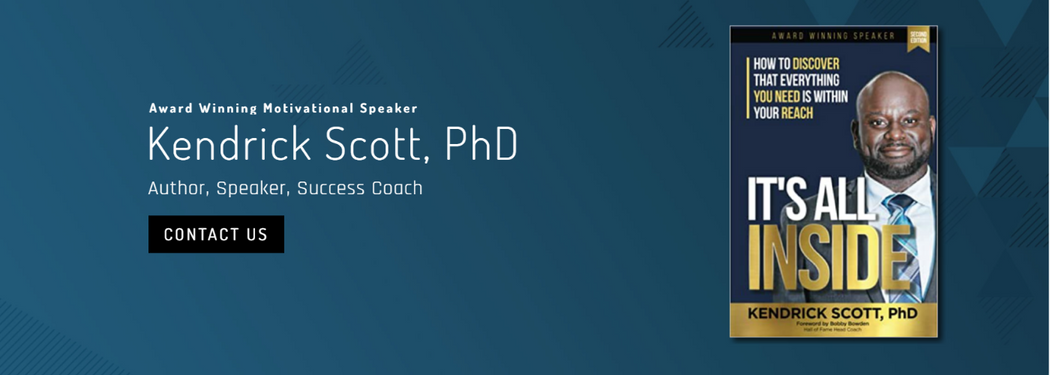
Predictive Maintenance
Predictive maintenance is a revolutionary approach in the operational strategies of various industries, leveraging data analytics to enhance efficiency and reduce operational downtime. This technique involves the use of data-driven insights to predict when an equipment failure might occur, allowing maintenance to be performed just in time to avert a breakdown. This is a significant shift from traditional maintenance strategies, which often rely on scheduled or reactive maintenance models.
The core of predictive maintenance is the utilization of sensors and IoT (Internet of Things) devices that collect data in real time from equipment. This data can include anything from vibration, temperature, pressure, or sound levels, depending on the type of machinery. Advanced analytics and machine learning algorithms analyze this data to detect patterns or anomalies that precede equipment failures.
By implementing predictive maintenance, companies can significantly extend the lifespan of their machinery, reduce maintenance costs, and minimize unplanned downtime. This not only improves operational efficiency but also boosts overall productivity. The ability to predict equipment failure has a direct impact on the bottom line, as it significantly reduces the amount of time and resources spent on emergency repairs and helps maintain a steady production flow.
Furthermore, predictive maintenance supports a more sustainable operational approach. By optimizing the timing of maintenance activities, it ensures that parts and equipment are only replaced or repaired when necessary, reducing waste and promoting more efficient use of resources. This operational efficiency extends beyond cost savings, enhancing a company’s reputation for reliability and forward-thinking in managing its assets and operations.
Real-Time Data Monitoring
Real-Time Data Monitoring plays a crucial role in enhancing operational efficiency across various industries. By leveraging data analytics, businesses can continuously observe and analyze data as it is generated, allowing for immediate responses to any operational issues or opportunities. This capability is vital in environments where conditions change rapidly, such as manufacturing, logistics, and e-commerce.
The implementation of real-time data monitoring systems enables companies to detect inefficiencies and bottlenecks as they occur. For instance, in a manufacturing setting, sensors can monitor production lines to ensure equipment operates within specified parameters. If a deviation occurs, such as a drop in the speed of an assembly line or an unexpected temperature increase, the system can alert managers or trigger automated adjustments. This immediate response helps to minimize downtime, reduce waste, and maintain the quality of the output.
Furthermore, real-time data monitoring supports decision-making processes. With up-to-the-minute data, managers can make more informed decisions that reflect the current state of business operations. This agility is particularly important in fast-paced sectors where delayed decisions can lead to missed opportunities or escalated problems.
Additionally, this approach aids in resource management. By understanding the real-time demand and performance data, businesses can optimize the allocation of resources, whether it be human labor, machinery, or materials. This optimization not only reduces costs by avoiding overuse of resources but also ensures that no part of the operation is under-resourced, thus maintaining operational flow and preventing bottlenecks.
In summary, real-time data monitoring, facilitated by advanced data analytics, is indispensable for modern businesses aiming to maintain high operational efficiency. It provides the tools necessary for proactive management and agile response capabilities, which are essential in today’s dynamic market environments.
Process Optimization
Process optimization is a crucial aspect of enhancing operational efficiency through data analytics. It involves systematically refining business processes to improve efficiency, quality, or performance. By leveraging data analytics, organizations can gain deep insights into their operations, identifying inefficiencies and potential improvements in their processes.
The application of data analytics in process optimization typically starts with the collection and analysis of large volumes of operational data. This data can come from various sources, including production equipment, supply chain management systems, and customer feedback platforms. Analytical tools and techniques, such as statistical analysis, machine learning, and predictive modeling, are then used to interpret this data. These tools help in uncovering patterns, trends, and correlations that might not be obvious through manual observation.
One of the key benefits of using data analytics for process optimization is the ability to identify bottlenecks in the production or service delivery processes. For instance, data analysis can reveal that a particular production stage consistently takes longer than others, suggesting a need for process re-engineering or resource reallocation to balance the workflow. Furthermore, analytics can aid in determining the optimal set of conditions under which machines and systems perform most efficiently, thereby reducing waste and increasing throughput.
Moreover, process optimization through data analytics facilitates better decision-making. Armed with accurate and timely data, managers and decision-makers can make more informed choices about process adjustments. This capability enables businesses to be more agile, adapting quickly to changes in market conditions or internal production dynamics.
In summary, process optimization using data analytics is about making smarter, data-driven decisions to streamline operations, enhance productivity, and ultimately achieve higher levels of operational efficiency. By continuously analyzing and refining processes, companies can maintain competitive edges, reduce costs, and improve overall performance.
Supply Chain Management
Supply Chain Management (SCM) plays a critical role in enhancing operational efficiency through the application of data analytics. By integrating data analytics into SCM, organizations can significantly improve their decision-making processes, optimize inventory levels, reduce costs, and enhance overall supply chain performance.
Data analytics enables businesses to analyze vast amounts of data from various sources within the supply chain, such as supplier quality data, transportation data, and customer demand forecasts. This analysis helps in identifying patterns, predicting trends, and making informed decisions. For instance, predictive analytics can be used to anticipate product demand, allowing companies to adjust their production schedules and inventory levels accordingly. This not only helps in meeting customer demand more accurately but also reduces the costs associated with overproduction and excess inventory.
Furthermore, data analytics can improve the responsiveness of the supply chain by enabling real-time data monitoring. This allows companies to quickly detect and respond to supply chain disruptions, such as delays in supplier shipments or sudden increases in demand. By having a more agile supply chain, companies can minimize downtime and improve their customer service levels.
Additionally, data analytics contributes to better supplier management by providing insights into supplier performance and risk. Companies can use these insights to negotiate better terms, select the best suppliers, and manage risks more effectively. This is crucial in maintaining a stable and reliable supply chain that can adapt to changes and challenges.
Overall, the integration of data analytics into supply chain management not only boosts operational efficiency but also provides a competitive edge in today’s fast-paced market environment. Companies that effectively harness the power of data analytics in their supply chains can expect to see significant improvements in efficiency, cost savings, and customer satisfaction.
Customer Experience Analysis
Customer Experience Analysis is a critical aspect of operational efficiency, particularly in service-oriented industries. By analyzing data related to customer interactions, preferences, and feedback, businesses can gain valuable insights into what drives customer satisfaction and loyalty. This information can then be leveraged to refine products, services, and processes, ultimately enhancing the overall customer experience.
One of the primary benefits of Customer Experience Analysis is the ability to identify patterns and trends in customer behavior. For instance, data analytics can reveal which features of a product or service are most appreciated by customers, or which aspects lead to customer dissatisfaction. Armed with this knowledge, a company can make targeted improvements to increase the value they deliver to their customers, thereby increasing their competitive advantage.
Furthermore, Customer Experience Analysis helps in personalizing interactions and offerings to meet the specific needs and preferences of individual customers. This personalized approach not only improves customer satisfaction but also increases customer retention rates. For example, by analyzing browsing and purchase history, a company can recommend products that a customer is likely to be interested in, thereby enhancing the shopping experience.
In conclusion, Customer Experience Analysis is a pivotal tool for businesses seeking to enhance operational efficiency. By providing a deep understanding of customer needs and behaviors, it enables businesses to make informed decisions that improve service quality, customer satisfaction, and loyalty, all of which are essential for long-term success and profitability.
Leave a Reply